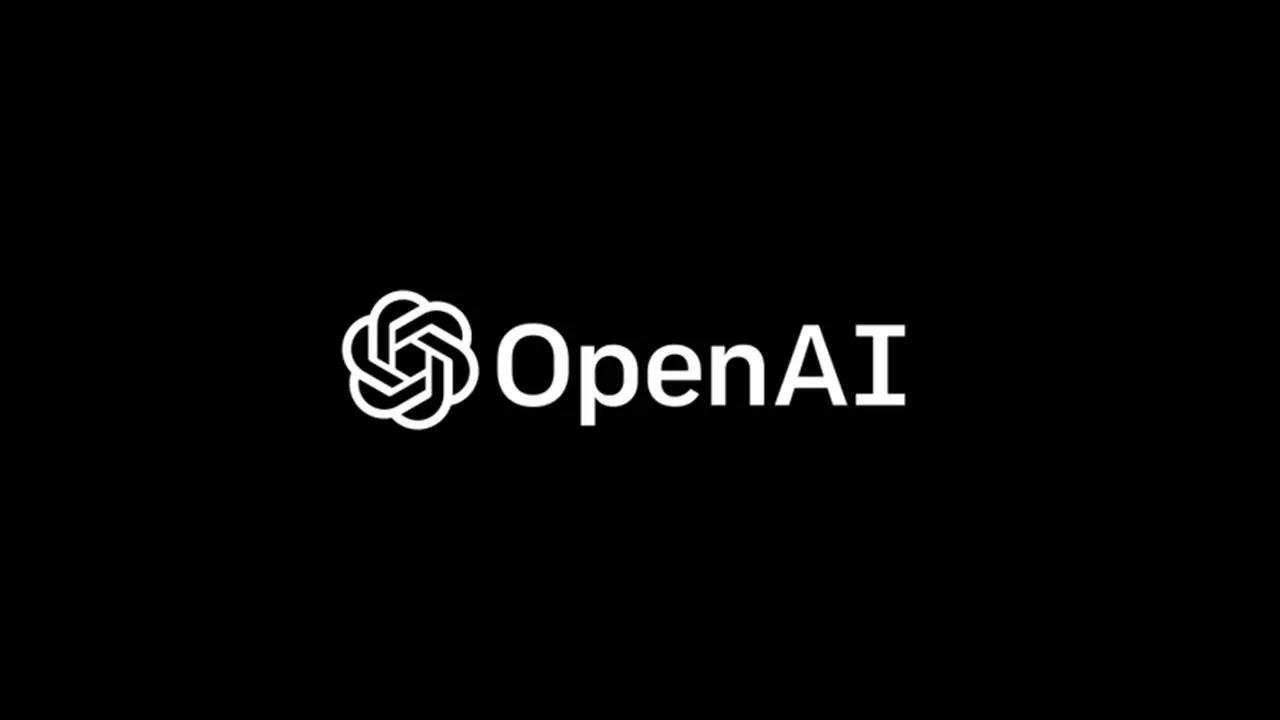
Microsoft Invested Billions in ChatGPT Maker OpenAI — So, Who Owns It?
OpenAI, the maker of the AI tool ChatGPT, is owned by a group of technology entrepreneurs. It has received multiple investments from Microsoft.
Market Realist has the latest news and updates on the stock market and trending stocks.