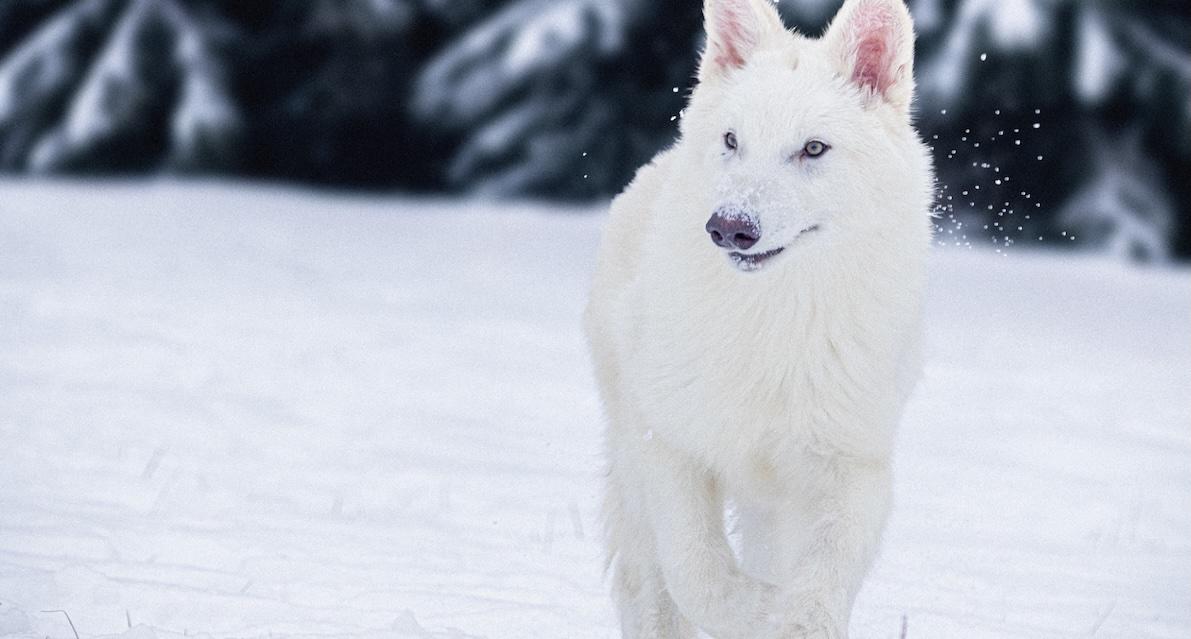
Colossal's Dire Wolves Mark a Biotech Milestone
The biotechnology industry reached a watershed moment in 2025 with the successful birth of Colossal Biosciences' dire wolves.
Market Realist has the latest news and updates on the stock market and trending stocks.