Take a Look at the AI Technology That Can Effectively End Medicare Fraud
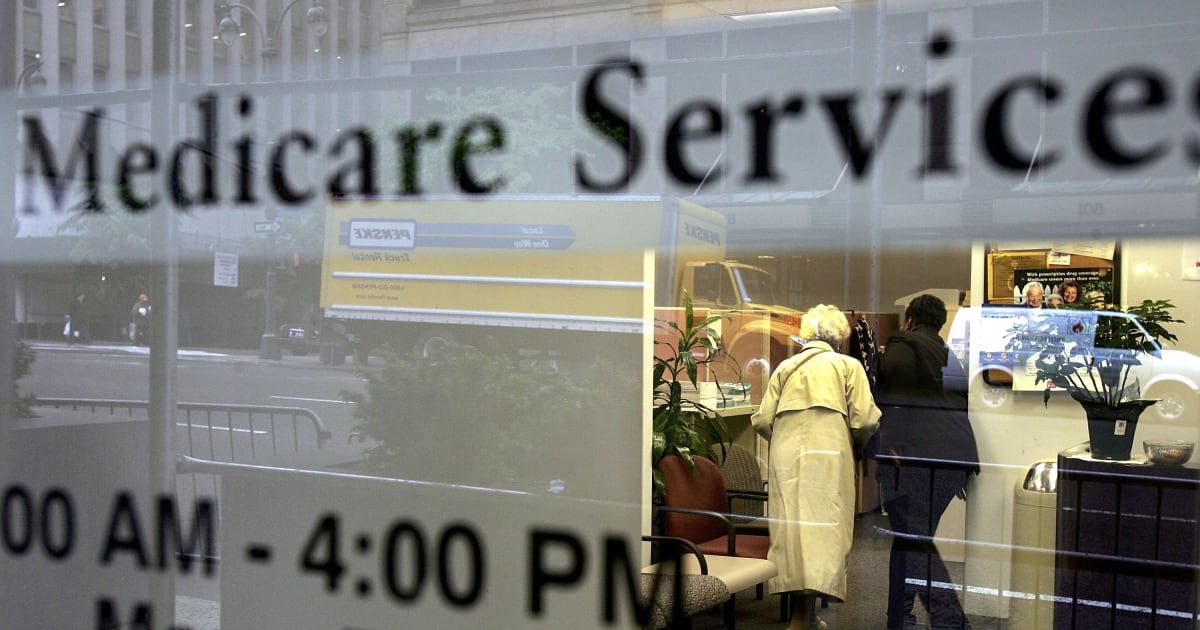
Medicare, an important healthcare support system, faces a continuous threat from fraudulent insurance claims, and with an estimated annual loss of $100 billion to fraud, innovative solutions are the need of the hour. Florida Atlantic University's College of Engineering and Computer Science tackles this challenge head-on; their groundbreaking approach zeroes in on fraudulent activities within Medicare. By quickly identifying instances of fraud, the AI system serves as an essential step in preventing fraudulent practices, potentially saving significant resources for the Medicare system.
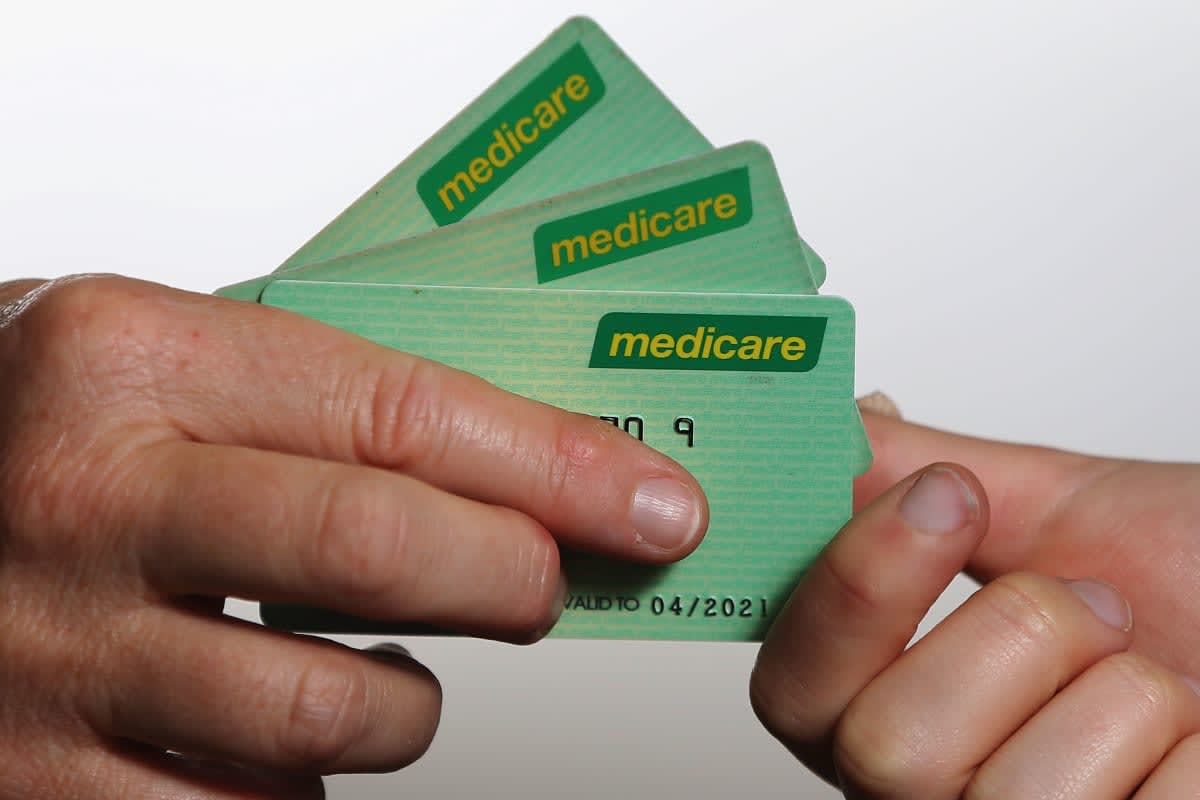
Traditional methods of fraud detection involve a limited number of auditors manually inspecting thousands of claims, struggling to identify specific patterns indicative of suspicious behavior. The rise in medicare scams emphasizes the urgency for advanced detection mechanisms. Researchers at Florida Atlantic University explored the complexities of Medicare insurance fraud detection, leveraging big data analytics to tackle imbalanced datasets and high dimensionality challenges.
The research introduces two key techniques, namely Random Undersampling (RUS) and supervised feature selection. RUS involves strategically removing samples from the majority class, achieving a balance between minority and majority classes. On the other hand, the supervised feature selection method relies on feature ranking lists. To establish a benchmark, models were constructed using all features available in the datasets. With the finalized ranking in hand, features were chosen based on their respective positions within the list.
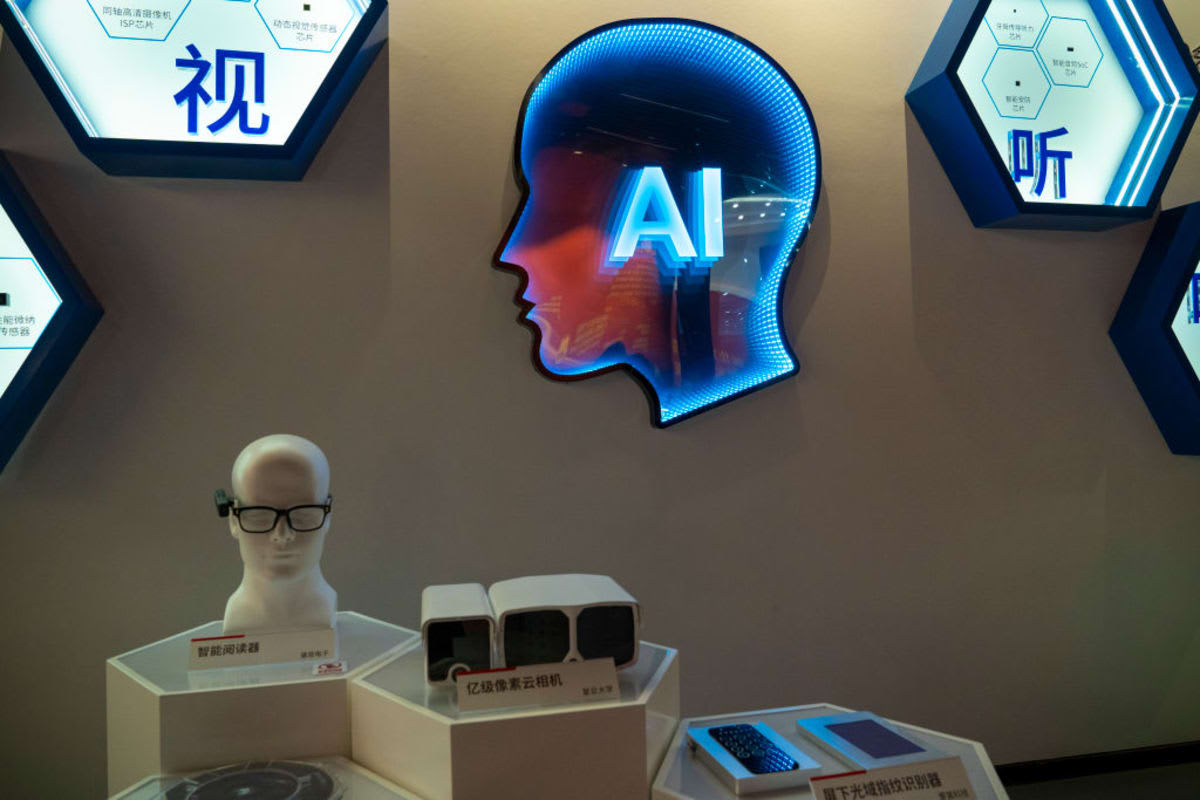
The experimental design explores various scenarios, highlighting the synergy between the two techniques. Results demonstrate that intelligent data reduction techniques significantly improve the classification of the imbalanced data available for Medicare. Moreover, the combination of RUS followed by feature selection or vice versa managed to outperform other models that used all available features.
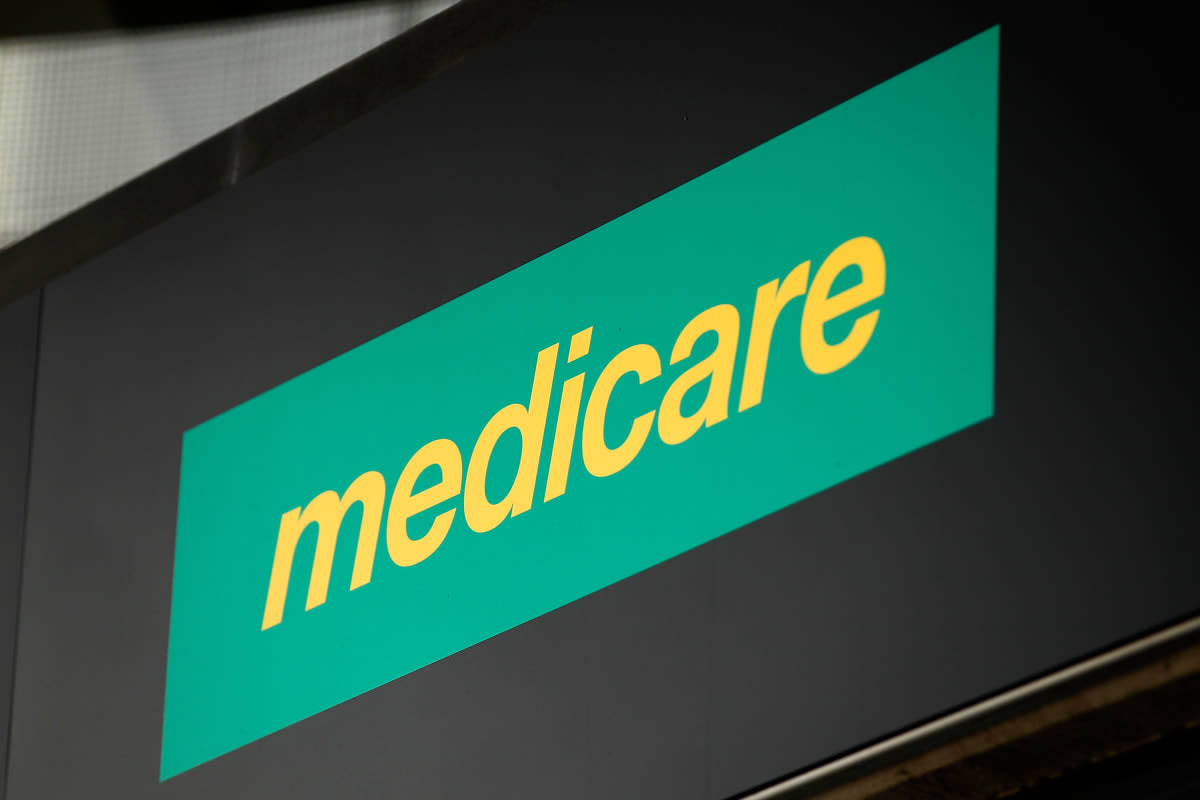
With Medicare fraud posing a severe threat to the healthcare system, this research not only offers computational advantages but also enhances the effectiveness of fraud detection systems. By reducing the number of features, models become more explainable, facilitating a deeper understanding of fraudulent activities. It also increases the scope of people that the data can ve communicated to, in order to achieve a higher level of awareness against fraud.
Dr Taghi Khoshgoftaar, the senior author and Motorola Professor at FAU emphasizes the significance of their systematic approach in comprehending the interplay between feature selection and model strength. This method provides a clearer understanding of how models perform classifications when built with fewer features.
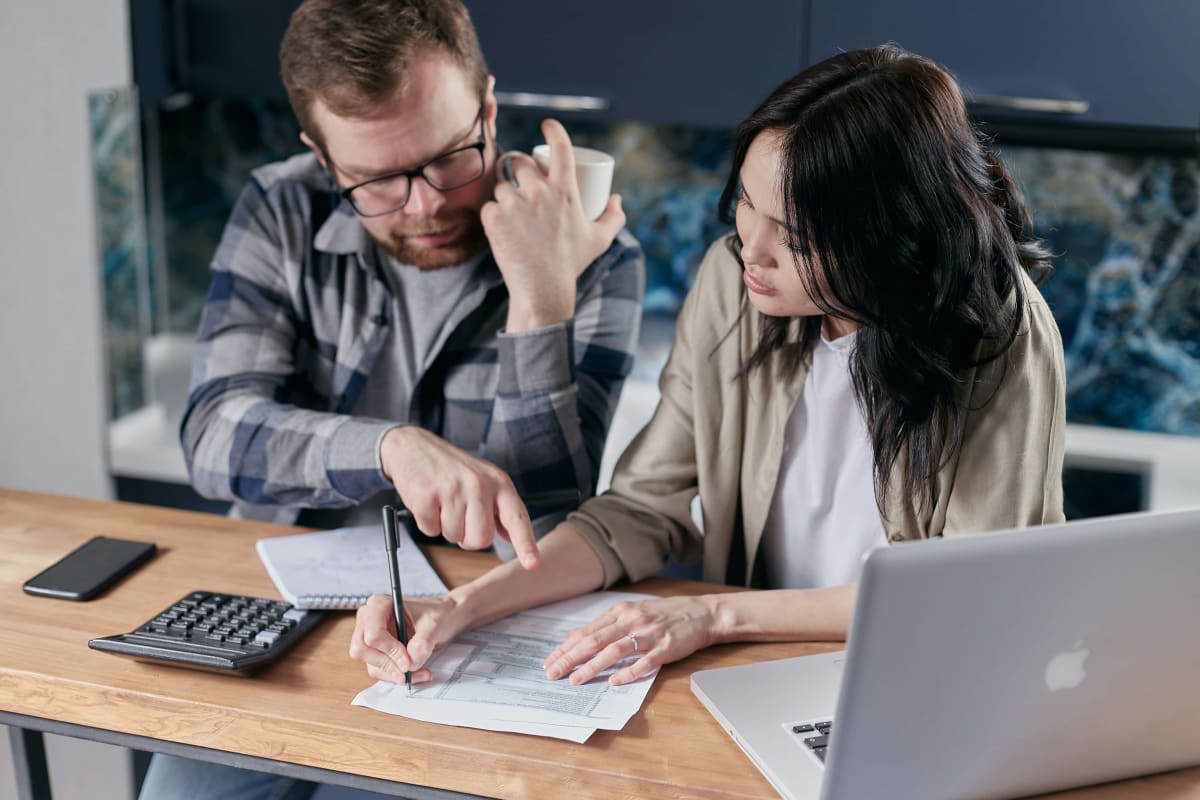
Dean Stella Batalama, Ph.D., dean, FAU College of Engineering and Computer Science, states, "Given the enormous financial implications of Medicare fraud, findings from this important study not only offer computational advantages but also significantly enhance the effectiveness of fraud detection systems." "These methods, if properly applied to detect and stop Medicare insurance fraud, could substantially elevate the standard of health care service by reducing costs related to fraud," he added.
As Florida Atlantic University's research marks a transformative leap in Medicare fraud detection, the healthcare industry continues to grapple with the financial implications of fraud. However, these advancements promise to enhance computational efficiency and overall effectiveness in safeguarding the integrity of healthcare services.