CEO Lisa Su: AMD to Increase Artificial Intelligence Profile
The Hot Chips 31 Symposium started this week. Artificial intelligence was the hot topic among participants Intel, NVIDIA, and Advanced Micro Devices.
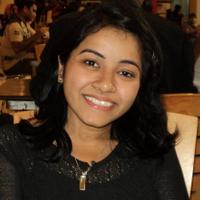
Aug. 22 2019, Published 6:14 p.m. ET
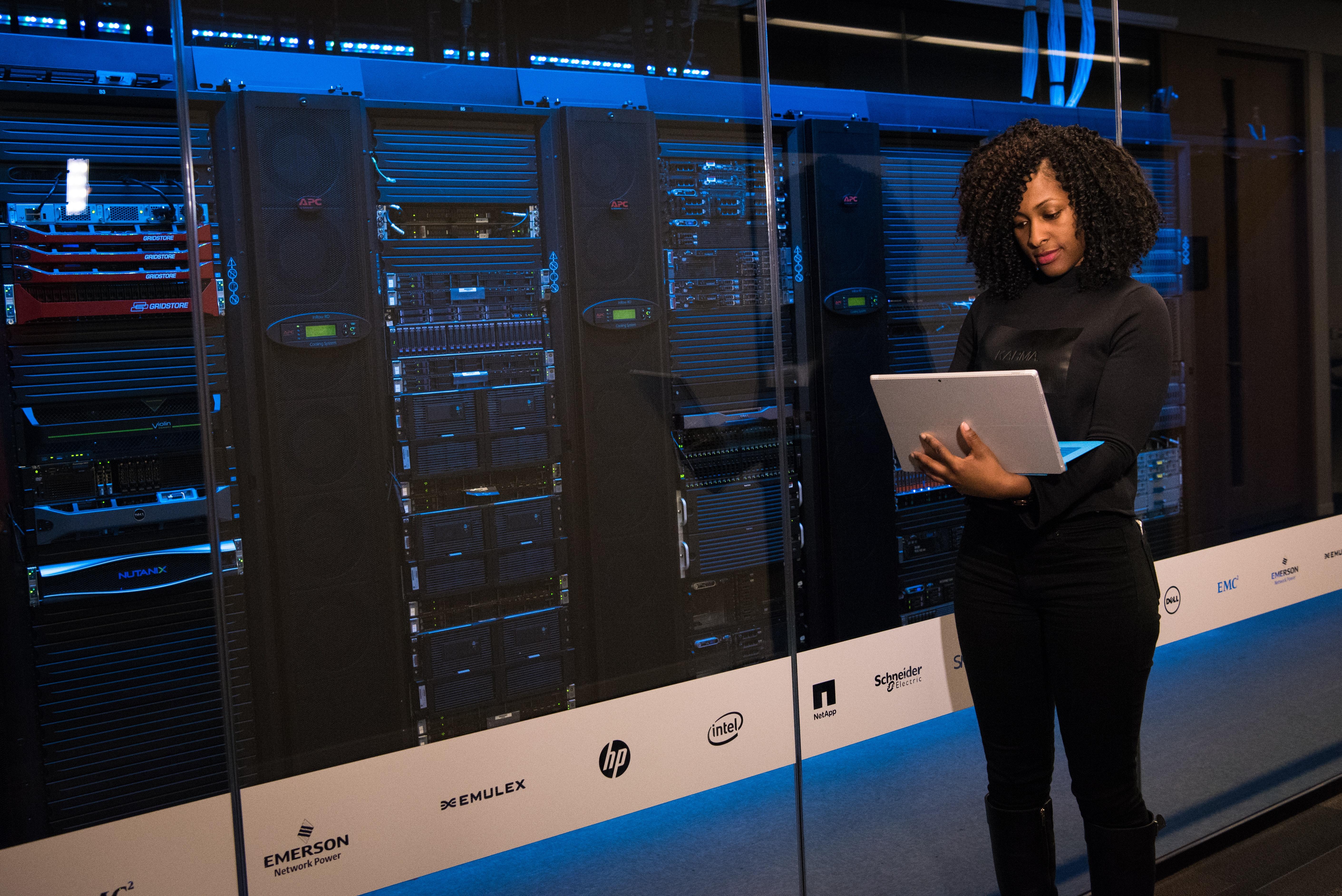
The Hot Chips 31 Symposium started this week. AI (artificial intelligence) was the hot topic among participants Intel (INTC), NVIDIA (NVDA), and Advanced Micro Devices (AMD). The stocks of these three chip companies rose 1.18%, 2%, and 3.2%, respectively, on August 21 as they discussed their AI strategies.
It is essential for long-term investors to understand the three companies’ AI opportunity. All three have a different approach to tapping AI, so their AI TAM (total addressable value) is also different. What remains to be seen is which company’s strategy works out best for investors.
Hot Chips 31: Intel’s, AMD’s, and NVIDIA’s artificial intelligence strategy
NVIDIA estimates its data center artificial intelligence TAM to reach $50 billion by 2023. This comprises HPC (high-performance computing), DLT (deep learning training), and DLI (deep learning inference).
Intel estimates its DLT and DLI TAM to reach $46 billion in 2020. AMD has not released any TAM for deep learning, as it is more focused on gaining market share from Intel and NVIDIA. So, AMD does not have an artificial intelligence–focused chip. However, AMD CEO Lisa Su stated that the company is working toward becoming a more significant player in artificial intelligence.
Lisa Su: CPU computing has its limitations
Any computing performance discussion starts with Moore’s law, which is slowing. Moore’s law states that computing performance will double every two years as chip sizes shrink and transistor density increases.
Lisa Su, in a keynote address at Hot Chips 31 reported by AnandTech, explained that companies have been improving their CPU (central processing unit) performance by leveraging various elements. These elements are process technology, die size, TDP (thermal design power), power management, microarchitecture, and compilers.
Process technology is the biggest contributor, as it boosts performance by 40%. Increasing die size also boosts performance in the double digits, but it is not cost-effective.
AMD used microarchitecture to boost EPYC Rome server CPU IPC (instructions per cycle) by 15% in single-threaded and 23% in multi-threaded workloads. This IPC improvement is above the industry average IPC improvement of around 5%–8%. However, all the above methods double performance in 2.5 years.
Su: Accelerated computing needed for artificial intelligence
Su stated that on the one hand, Moore’s law is slowing. On the other hand, the performance of the world’s fastest supercomputers is doubling every 1.2 years. This means the solutions of the past decade won’t work.
The industry’s current need is to optimize parts of the system to make them ideal for artificial intelligence workloads. She explained that performance per watt is the highest in ASICs (application-specific integrated circuit) and FPGAs (field-programmable gate arrays) and the lowest in CPUs. General-purpose GPUs (graphics processing units) fall between CPUs and FPGAs in performance per watt.
Su stated that every artificial intelligence workload has a different computational requirement. Interconnect technology is the solution, as it interconnects various parts to a single system. She explained this point with the following examples:
- NAMD (Nanoscale Molecular Dynamics) workload depends on GPU
- NLP (natural language processing) workload is balanced across CPU, GPU, memory bandwidth, and connectivity
The industry has improved CPU and GPU performance using traditional methods. Su highlighted that the industry should improve performance by focusing on interconnect, I/O (input/output), memory bandwidth, software efficiency, and software-hardware co-optimization.
Su: AMD will be a larger player in artificial intelligence
AMD’s CEO stated that the company had adopted a CPU/GPU/interconnect strategy to tap artificial intelligence and HPC opportunity. She said that AMD would use all its technology in the Frontier supercomputer. The company plans to fully optimize its EYPC CPU and Radeon Instinct GPU for supercomputing. It would further enhance the system’s performance with its Infinity Fabric and unlock performance with its ROCM (Radeon Open Compute) software tools.
Unlike Intel and NVIDIA, AMD does not have a dedicated artificial intelligence chip or application-specific accelerators. Despite this, Su noted, “We’ll absolutely see AMD be a large player in AI.” AMD is considering whether to build a dedicated AI chip or not. This decision will depend on how artificial intelligence evolves.
Expanding her point, Sue added that many companies are developing different artificial intelligence accelerators like ASICs, FPGAs, and Tensor Processing Units. These chips will narrow to the most sustainable chips, and then AMD will decide whether to build that widely used accelerator.
In the meantime, AMD will work with third-party accelerator makers and connect their chips with its own CPU/GPU via its Infinity Fabric interconnect. This strategy is similar to its ray-tracing strategy. NVIDIA introduced real-time ray tracing last year, but AMD did not rush to launch this technology. Instead, Su stated that AMD would introduce ray tracing in the future when the ecosystem is in place and the technology is widely adopted.
Given the fact that AMD is a smaller player competing with larger players with ample resources, the above strategy makes economic sense. Tapping share in an already established market mitigates the risk of product failure due to poor adoption and guarantees minimum returns.
AMD’s AI strategy differs from that of Intel and NVIDIA 
AMD is adopting a wait-and-see approach before developing its own AI chip. Instead, it is leveraging its existing technology for AI workloads.
On the other hand, Intel is developing every element of computing performance in-house. It has developed its Xeon CPU, Optane memory, Altera FPGA, and interconnect. It is also developing its own Xe GPU. At Hot Chips 31, Intel unveiled its dedicated Nervana AI chips for DLT and DLI. Intel manufactures its chips in-house. While this gives Intel greater control over its technology, it requires significant time and resources.
NVIDIA’s AI strategy is to supply general-purpose GPUs along with CUDA software support that can be used in any AI application. It also has an NVL ink interconnect. The company is exploring new markets for artificial intelligence by partnering with enterprises. Although this strategy involves a lot of research and risk of failure, these risks give good rewards in the form of high returns.