Can Intel Compete with NVIDIA in the AI Space?
For the last few years, Intel (INTC) has been shifting its focus away from PC to data-centric businesses. It’s looking to tap future technologies such as AI.
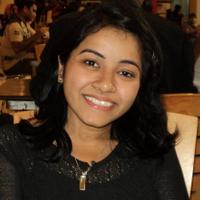
Aug. 28 2019, Published 10:06 a.m. ET

For the last few years, Intel (INTC) has been shifting its focus away from PC to data-centric businesses. It’s looking to tap future technologies such as AI, autonomous vehicles, and 5G networking infrastructure. NVIDIA (NVDA) is a leader in the AI space. Intel has identified NVIDIA as its AI competitor, as data centers prefer the latter’s Tesla GPUs (graphics processing unit) for their AI workloads. Intel has tried to compete with NVIDIA’s Tesla GPUs with its Altera field-programmable gate arrays, Xeon Phi processors, and traditional Core processors.
To accelerate its efforts in the AI space, Intel invested in Israeli AI startups Habana Labs and NeuroBlade and acquired AI startup Nervana Systems in 2016. At the Hot Chips symposium, Intel announced its first AI-powered Nervana NNPs (neural network processor): NNP-T for training and NNP-I for inference. Despite having its own fabrication facilities, Intel is building these NNPs on TSMC’s (TSM) 16 nm (nanometer) CLN16FF+ processor. It’s doing so because Nervana Systems had already started manufacturing NNP chips on TSMC’s node before Intel acquired it. Any shift in the fabrication facility could lead to product delays, poor yields, and weak product quality. Hence, Intel decided to continue with TSMC.
Intel in deep learning training and inference
Until now, Intel was optimizing its existing general-purpose Xeon CPUs (central processing unit) for DL (deep learning) training and inference. This technique wasn’t efficient, so it developed dedicated processors to provide flexibility and efficiency in various types of DL models.
Intel’s NNP-T (code-named Spring Crest) is a scalable 16 nm processor featuring 24 tensor cores dedicated to AI workloads. Even NVIDIA’s Volta and Turing GPUs and Google’s custom tensor processing unit use tensor cores for AI. NNP-T features 32 GB of HBM2 (high-bandwidth memory) and delivers the performance of 119 TOPS (theoretical operations per second). The CPU supports an x16 PCIe 4.0 (peripheral component interconnect express) connection and is expected to consume 150–250 watts of power. Nervana Systems connects all these elements on a single die using TSMC’s advanced chip-on-wafer-on-substrate packaging.
Intel’s NNP-T balances memory, processors, and networking, which enables it to train a network on larger models and datasets within a given power budget. NNP-T has four-lane quad small form-factor pluggable network ports, which enable users to connect multiple NNP-T chips together on the same chassis. NVIDIA also offers such a feature, with which consumers can connect multiple Tesla GPUs.
Unlike NNP-T, which was built from scratch, NNP-I (code-named Spring Hill) is a modified 10 nm Ice Lake processor. NNP-I features 12 inference compute engines that support various instruction formats, and it offers a high degree of programmability. It has four 64 GB LPDDR4x (low-power dynamic random-access memory) modules for high-speed memory bandwidth. It consumes 10–50 watts of power and supports PCIe 3.0 and 4.0. NNP-I delivers a performance of 4.8 TOPS per watt. Baidu is using NNP-T, and Facebook is using NNP-I. Intel hasn’t announced when it will launch its NNP chips.
NVIDIA still a leader in AI
Intel’s NNP chips are its first AI-powered chips. In contrast, NVIDIA has already moved ahead with its third-generation AI architecture, Turing (after Pascal and Volta). The Turing GPU includes Tensor cores for AI and DL workloads and RT Cores for ray tracing and rendering. NVIDIA is also reporting key milestones in AI and DL.
According to an NVIDIA blog post on August 13, “The NVIDIA DGX SuperPOD with 92 DGX-2H nodes set a new record by training BERT-Large [bidirectional encoder representations from transformer] in just 53 minutes.” Bert-Large is the world’s largest transformer-based language model. The trained model was able to infer in just over two milliseconds compared to the industry’s ten milliseconds. Intel has a long way go toward building AI-powered chips that can actually compete with NVIDIA’s GPUs.
Can AMD compete with Intel in AI?
While Intel has already moved forward in the AI space with dedicated NNP chips, AMD is looking to tap the AI opportunity. On the one hand, AMD is moving ahead of Intel in the traditional x86 CPU market. On the other hand, AMD is behind Intel in terms of its future-generation AI technology. AMD has a long way to go to gain ground in the AI space, but it has the technology and capability to become a key player. Right now, AMD competes with Intel in the CPU market and NVIDIA in the GPU market. In the future, we could see AMD compete with Intel and NVIDIA in the AI market.